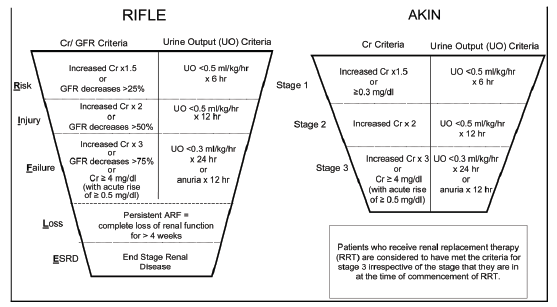
Figure 1: Correlation between the Relative Blood volume and the pulse rate (r=-0.4, p=0.03)
Tania khan1 Jonathan Williams1 Ashwinkumar Patel2 Ahmed Mattar1 Anjit Khurana1 Daniela Johnson1 Ankit Patel1 Amr Takieldeen1 David Carlson1,3 Adil Ahmed1,4*
1North Central Texas Medical Foundation, Wichita Falls Family Practice Residency Program, Wichita Falls, Texas, USA*Corresponding author: Adil Ahmed, Institute for Health Informatics, University of Minnesota, Minneapolis, Minnesota, USA, Tel: 507-269-1799; E-mail: aahmed@wfresidency.org
Timely recognition and treatment of specific critical care syndromes are the key determinants of outcomes of critical illness, regardless of the underlying cause. Acute kidney injury (AKI) is a prototypical example of a major health problem for which the efficacy of treatment is already limited once the condition is fully established. The condition is rarely present at the time of hospital admission, but develops within hours or days after an initial insult. Due to this delay in diagnosis, both therapeutic and experimental interventions are currently instituted late in the course of the illness, which limits the potential for therapeutic impact.
Timely recognition of patients at risk of AKI would allow for novel and potentially more meaningful therapeutic and preventative strategies. Advances in medical informatics and widespread implementation of electronic medical records provide the opportunity to improve early recognition and treatment of specific critical care syndromes. In this study, we plan to develop a predictive model of AKI using probabilistic modeling. If successfully validated, this model will allow for early recognition of patients at risk of AKI for the purpose of diagnosis, prognosis, and future prevention trials.
Acute kidney injury; Electronic medical records; Modeling
Acute Kidney Injury (AKI) is one of the most prevalent clinical complications among patients admitted to an intensive care unit (ICU). AKI incidence in the ICU has been reported to range from 5 to 80% [1]. Morbidity and mortality due to AKI correlates with the severity of renal dysfunction [2]. AKI is also an independent risk factor for the development of chronic kidney disease and end-stage renal disease [3].
Over the last several decades, there has been significant confusion regarding the terminology used to describe renal failure. A systematic review conducted in the early nineties showed that in 24 out of 28 studies, no two studies used the same definition for renal failure [4]. This definitional ambiguity hindered researchers’ understanding of the true impact of this disease in critically ill patients and impeded epidemiologic and incidence studies [5]. In 2004, the Acute Dialysis Quality Initiative (ADQI) group proposed a standardized definition for the syndrome. AKI was defined by the risk of renal failure, injury to the kidney, failure of kidney function, loss of kidney function, and end-stage renal failure (RIFLE) [6]. In 2007, to increase the sensitivity and overcome some of the limitations of the RIFLE criteria, AKI definitions were modified by the Acute Kidney Injury Network (AKIN) group [7] (Figure 1).
Figure 1: Correlation between the Relative Blood volume and the pulse rate (r=-0.4, p=0.03)
The increased acceptance of standardized definitions (RIFLE and AKIN) of AKI by the scientific and medical communities has marked a new era in the study of AKI. These definitions have been used in the vast majority of recently published studies, with various objectives and outcomes [2]. We are now at the point where we can strive to predict the development of AKI.
Early diagnosis of AKI can potentially provide a wider therapeutic window for both prophylaxis and treatment of AKI and its related complications. Diagnosis of AKI at the time of hospital admission could decrease the chance of patients suffering a “second hit” from omissions and delays in medical interventions. This, in turn, may decrease morbidity and mortality associated with AKI [8]. Therefore, the overall objective of this protocol is to develop and validate an automated electronic kidney injury clinical prediction model that can be integrated in the electronic medical records.
Using data available in the electronic medical records (EMR) system, the first aim is to retrospectively develop and validate a rule-based electronic algorithm to identify patients with hospital-acquired AKI, defined as AKI development more than 12 hours after hospital admission, using the AKI criteria.
Our second aim is to electronically identify at-risk patients for AKI development at the time of hospital admission, based upon the clinical predication model validated in Specific Aim 3. So doing will facilitate enrollment of patients into mechanistic studies, as well as future AKI prevention trials,
To prospectively validate a clinical prediction model using an EMR syndrome surveillance tool for identifying patients at high risk of developing AKI in an independent cohort of consecutive hospitalized patients.
To facilitate enrollment of patients into future mechanistic studies and AKI prevention trials, we will retrospectively identify patients with hospital-acquired AKI.AKI outcomes will be used to develop a prediction model using the appropriate risk factors. Risk factors will be determined at the time of hospital admission and all potential risk factors will be entered into the tool. Finally, the model will be automated and validated prospectively in a sample population.
To develop and validate a rule-based electronic algorithm, to detect patients with hospital acquired AKI in subset of ICU patients.
Both derivation and validation cohorts will be adults’ ≥ 18 years of age and admitted to an ICU hospital during the year 2015. Exclusion criteria are listed in (Table 1).
For SA#1, an electronic algorithm will be developed to predict development of AKI. The effectiveness of this algorithm will be compared to two search strategies; manual searches and searches using ICD-9 codes. Patient eligibility will be reviewed by two blinded investigators, who will ascertain the presence of AKI using the AKIN criteria [7]. Disagreements will be resolved by a third reviewer. As reported in other studies, baseline Creatinine will be defined as the median value of Creatinine between 6months and 7 days prior to hospital admission. For those patients with no record of a serum Creatinine in the EMR in the 6 months prior to ICU admission, we will use the revised Modification of Diet in Renal Disease (MDRD) formula [9]. AKIN working group staging criteria will be used to divide the outcome into three stages. Hospital-acquired AKI will be defined as the onset of AKI in a hospitalized patient who either had no evidence of AKI (i.e., reduced urine output or elevated serum creatinine) at the time of hospital admission, or in whom AKIN criteria were satisfied within 12 hours of hospital admission.
Manual data will be considered as a reference standard and will be divided into two datasets: derivation and validation cohorts. Data from the derivation cohort will be used to develop and refine the electronic algorithm by reviewing all false positive and false negative cases, compared to manually-ascertained cases of AKI. For ICD-9 codes, we will be using data from the hospital administrative database to identify ICD-9 diagnosis codes for acute renal failure. The codes to be used are listed in the Appendix (Table 1).
Table 1: Exclusion criteria
Primary outcomes will be the sensitivity and specificity for each search strategy in both the derivation and validation cohorts, between the two strategies. Positive (PPV) and negative predictive values (NPV) will be calculated along with percentage agreement and Cohen’s kappa statistics.
Secondary outcomes will be the time to AKI between electronic and manual strategies in the validation set. Time will be defined as the time between admission and the first time AKIN criteria are met. It, along with the firing criteria (Urine Output vs. serum creatinine), will be compared between the two methods.
We will develop and validate a clinical prediction model for identifying patients at high risk of developing AKI at the time of hospital admission. We will create a rule-based method for capturing electronic data, including baseline clinical, demographic, and environmental exposure information that is available on the first day of hospital admission. This will include data about acute and chronic illnesses as well as data on interventions and outcomes (e.g., patients and systems) relating to AKI.
Eligible subjects will be consecutive hospital admissions of adult patients >18 yearsof age, who will have been admitted to community based hospital over a 10 year period (retrospective validation cohort, 2005-2014) with at least one predisposing condition at the time of hospital admission (e.g., sepsis, shock).
Demographic, clinical, and environmental predisposing conditions (Table 2) necessary for calculation of ecKLIPS will be collected based on the information present before or during the first 12 hours of hospitalization. The model will be developed using predisposing conditions and electronic algorithms that have been validated in previous studies [10,11]. For all other predisposing factors, an electronic search strategy will be developed and validated using methodology similar to the one in SA#1. All data will be extracted from the hospital electronic medical records and administrative databases.
Table 2: Suggested risk factors
Using the electronic tool developed and validated in the first Aim, we will identify all patients with AKI during their hospital stay. To derive the model, we will consider variables associated with the development or prevention of AKIin at least two prior published studies. Furthermore, a content expert will review the selected risk factors and rank them based on perceived importance. Hospital electronic medical records will provide datanecessary baseline and clinical information (e.g., demographics, clinical data, comorbid conditions, and laboratory data). Data collection will be accomplished by querying structured data from the EMR (e.g., laboratory data, vitals, and medication tables , ICD 9 data).All data will be restricted to 12 hours before AKI detection, unless specified otherwise. A sensitivity analysis will be performed using 6- and 24-hour time points. Multiple models will be developed using the derivation cohorts, different data points, and different time cutoffs.
During the derivation of the rule,we will include all independent predictors of AKI. Some of these predictors will be considered as predisposing conditions, the rest as modifier conditions, depending on the expert ranking. The magnitude by which each risk factor contributed to the development of AKI will be quantified according to the beta coefficients inour derivation cohort and the magnitude previously described for each factor in at least two prior studies.
To be included in the cohort, patients in the validation cohort have at least one predisposing condition. The variables needed to generate ecKLIPS for these patients will be collected retrospectively using automated search described earlier and by compiling information from the EMR and by trained investigators.
Our third aim is to prospectively validate a clinical prediction model using an EMR syndrome surveillance tool for identifying patients at high risk of developing AKI. Study subject and inclusion and exclusion criteria will be similar to those in SA#2.
The electronic surveillance tool built to detect at-risk patients will automate the ecKLIPS and run through patient records at 15-60 minutes resolution from admission to the emergency room until discharge, or when a patient meets AKI criteria. Over a three month period, an alert system using a threshold for high risk score will be prospectively implemented in selected medical floors and ICUs.
To identify patients at risk for AKI, an automated ecKLIPS model will run prospectively in the EMR databases and calculate the score to generate e-mail and/or a pager alert to a research coordinator when the score reaches the threshold for a high risk patient; a research coordinator will confirm and enroll the selected patients.
The primary outcome is development of AKI at any time during the hospital stay. Secondary outcomes will be the number of interventions in the alerted group, the proportion of patients who started renal replacement therapy, reduction of median serum creatinine at the time of hospital discharge, ICU and hospital mortality, and length of stay between the two groups.
All alerts will be collected in a separate electronic log, and the number of interventions will be assessed within three hours of the alert time. These will include ordering or administration of any of the following: fluids, defined as any bolus of 500 ml crystalloid or 250 ml colloid, diuretics,or vasopressors.
The purpose of Aim #1 is to validate that the data collected from the electronic algorithm areconsistent with the manually-collected data. Consistency will be assessed using sensitivity, specificity, positive, and negative predicted values.
The purpose of Aims#2 and #3 is to develop and validate a predictive model of AKI using a population-based sample. Using AKIN guidelines, we plan to model AKI using a penalized, logistic regression model, LASSO, to derive a parsimonious prediction rule. The explanatory variables will be derived using baseline patient risk factors, listed in Table 2. These factors are biologically-plausible and are recorded in the EMR. Serial laboratory risk factors will be converted to either the worst value within the first 12 hours of hospital admission, or by estimating the area under the curve for the first 12 hours. Measurements used but not taken within the first 12 hours will be considered missing. The “missingness” will be incorporated into statistical models with multiple imputation methods for the purposes of the sensitivity analysis.
The penalization parameter for the penalized, logistic regression will be determined using cross validation. Both receiver operating characteristic (ROC) curve and area under the curve (AUC) will be used to assess the prediction rule discrimination ability. Calibration of the scoring rule will be assessed using the Hosmer–Lemeshow test.
Boosted tree models will be compared to the penalized, logistic regression scorecard to predict AKI. The maximum number of trees used in the boosted model will be estimated using cross validation, depending on the shrinkage parameter and the number of splits from each tree. The boosted models classification will be compared to the penalized, logistic regression using the AUC from the test sample. Prediction rule performance measurements for the validation cohort will include AUC, sensitivity, specificity, negative predictive value, positive predictive value, and positive and negative likelihood ratios at specific thresholds of the score.
All data will be summarized as mean and standard deviation or median and interquartile range for continuous variables, and number and percentage for categorical variables and compared using appropriate statistical tests (i.e. t-test, chi square). P values will be two-tailed and will be considered statistically significant if p <0.05. SAS (SAS®, Cary, NC) and R (R Project for Statistical Computing, will be used for data analysis.
Nine possible patient enrollment scenarios for the minimal detectable AUC for the AKI prediction score shown in Table 3, assuming an alpha of 0.05 and 80% power [12]. Any sample where there are at least 500 cases and 500 controls can be adequately used for modeling.
Table 3: The minimal detectable AUC for the model, assuming an alpha of 0.05 and 80% power [38]
The actual AUC is expected to be larger than the minimally-detectible AUC. Table 4 shows seven possible validation patient enrollment scenarios, assuming an alpha of 0.05. To achieve a power of 0.80 there will need to be 200 to 400 patients enrolled, assuming 50% of cases are eligible, in the validation sample if the model fit AUC is 0.70 or larger and the expected validation sample AUC is expected to be greater than 0.60. The power calculation will be updated after the score has been developed.
Table 4: The power estimates assumed an AUC from the model fit, the number of cases and controls in the validation sample, and the null hypothesis for the AUC for the model applied to the validation set. Standard errors were estimated using the Obuchowski method [39].
In this study, we plan to develop a prediction model of AKI using probabilistic modeling. If successfully validated, this model would allow for early recognition of patients at risk of AKI for the purpose of diagnosis, prognosis, and future prevention trials.
Over the last three decades, a number of preventative strategies have been tested [13,14]. The overall classification of the types of prevention includes pharmacological and non-pharmacological venues [14].
Although many researchers have suggested that death and other AKI complications, like volume overload and electrolyte disturbances, modify mortality, these should be the primary goals for prevention. The fact is, some of the currently proposed strategies are usually applied as alternative or secondary interventions in the setting of a glomerular filtration rate (GFR) that has already declined [15]. Furthermore, despite the recent discovery of several biomarkers that may be useful for early diagnosis and prediction of AKI, their generalizability and clinical applicability have remained questionable [16].
Prevention models in critically ill patients have primarily focused on patients admitted to the ICU [17,18]. Unfortunately, the inferences from these studies with regard to AKI pathogenesis and potential preventative strategies are likely limited. By the time that AKI has been diagnosed in the ICU or during a hospital stay, the biological processes have already begun much earlier. In fact, the need for renal replacement therapy is frequently the very reason for ICU admission. This point has been reiterated multiple times in the literature [19,13].
Electronic medical records were introduced with the goal of improving the quality of care, increasing patient safety, and lowering medical costs [20]. Various models have been implemented successfully to increase compliance with evidence based medicine [21] and to improve quality of care [22]. Although technology facilitates proper documentation, it also permits faster and more accurate review of charts for the presence of complications and adverse events. Fitzhenry et al. [23] were able to reliably identify postoperative complications and adverse events in multiple veteran affairs hospitals using electronic tools. While the EMR allowed for more accurate and instantaneous decision support, models that used the EMR were usually limited by the quality of information contained within the system, and in many cases required a validation process [11].
There have been many models developed to identify patients at high risk for AKI. Since the standardization of AKI definitions, it has now become possible to validate and implement these models in different settings [17,24-28]. Depending on the outcome, some of these models have been used to predict mortality [24,29,30] while others have been used to predict the need for dialysis [31]. Furthermore, although multiple investigators have attempted detection of AKI, their approaches did not reach sufficient significance to modify patient outcomes [8]. For example, in a prospective study by Colpaertet al. [32] the use of the AKI sniffer through automation of the RIFLE criteria resulted in a significant number of interventions in the alert group. However, this approach did not impact other patient outcomes. The main reason for this was the small window for intervention in patients who already had injured kidneys [32].
Most medical mishaps, omissions, and waste occur during the early course of critical illness, when timely and efficient interventions are of vital importance for patient outcome. This intuitive concept, well-known as the “Golden Hours”, has informed trauma care since as early as four decades ago [33], but has yet to be widely adopted in most other critical care conditions. The nonlinear course and the time-sensitive nature of acute illness is characteristic of a complex system [34]. Avoidance of diagnostic errors and therapeutic delays during these first minutes and hours of the critical care process (“golden hours”) are necessary to prevent costly complications, preventable death, and disability [8,35].
The importance of timely recognition and appropriate treatment of acute critical illness is nicely illustrated by a multicenter quality improvement intervention targeting patients with severe sepsis in the emergency department. They observed that rapid implementation of early bundle elements (e.g., appropriate empiric antimicrobials, fluid bolus, and lactate) stopped progression of organ failure [36]. Furthermore, Balasubramanian et al. [37] showed that early involvement of a nephrologist in the care of patients with AKI can halt and reduce the risk of further decreases in kidney function. This confirmed the findings of an earlier study by Mehta et al. [8] in which the investigators found a significant increase in mortality associated with delays in consultation with a nephrologist [8]. It is anticipated that the model developed in this study will allow for even earlier identification of AKI which will subsequently allow for earlier intervention.
Following a demonstration of the model’s ability to discriminate such patients, additional steps will need to be taken to determine the clinical and bedside utility of the model as a decision support aid. Specifically, a multicenter validation of the electronic algorithms and the model predictability will be the most logical next step. However, the automation and testing of the final model in a real clinical environment needs to be carried out as a pilot study in a single center before it can be tested in a multicenter study.
The authors declare no conflict of interest
Download Provisional PDF Here
Article Type: Research Article
Citation: khan T, Williams J, Patel A, Mattar A, Khurana A, et al. (2016) Towards Prevention of Acute Syndromes: Development and Validation of an Automated Electronic Kidney Injury Clinical Prediction Score (Ecklips), Population-Based Protocol. Int J Nephrol Kidney Fail 2(3): doi http://dx.doi. org/10.16966/2380-5498.133
Copyright: © khan T, et al. This is an open-access article distributed under the terms of the Creative Commons Attribution License, which permits unrestricted use, distribution, and reproduction in any medium, provided the original author and source are credited.
Publication history:
All Sci Forschen Journals are Open Access