Figure 1: Adapted SIR model to form SIRD model-each compartment.
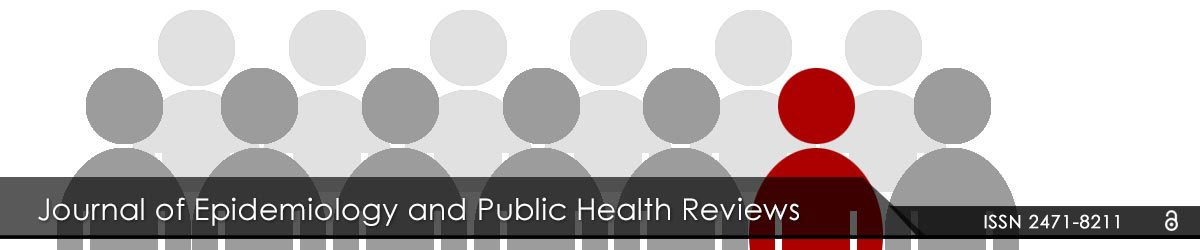
Full Text
Sofoora Kawsar Usman1*
Mariyam Suzana2
Sheena Moosa3
2Faculty of Health Sciences, Maldives National University, Maldives
3Research Centre, Maldives National University, Maldives
*Corresponding author: Sofoora Kawsar Usman, Ministry of Health, Male’, Maldives, E-mail: sofoora.kawsar@gmail.com
Background: Geo-spatial aspects affect the susceptibility and containment of infectious disease transmission. Small island geographies may make containment easy, but effective quarantine and isolation may be challenging due to different population density and living conditions. The mathematical models used in this paper aim to determine the epidemic dynamics of COVID-19, quantify the impact of the non-pharmaceutical interventions (NPIs) and forecast infection parameters in two types of island settings. The effects of the NPIs on the capital island and other are modelled islands separately.
Methods: The Susceptible, Infectious, Recovered, Deceased (SIRD) model is used to forecast the spread of COVID-19 in the dense and congested urban capital island compared with the less populated small island of the Maldives. The model parameter values were informed by published materials and fitted to the Maldives.
Results: The model shows that the reproduction number R0 , ranged between 1 and 2 in all the NPI states, and R0 could be decreased to less than 1 with combined interventions (NPI3-NPI5). The effects of interventions are particularly effective in smaller islands, and possible to bring down R0 to less than one even with minimal interventions. The densely populated capital city, Male’ will require double the time and double the combinations of NPIs to that required by other smaller islands to reach R0 below 1.
Conclusions: Epidemic modelling has greater utility for policy and planning when modelled for different populations separately rather than for the whole population especially when there are many geographically dispersed islands in the country, such as those in a number of Small Island Developing States. Findings from this model suggest that each community needs a unique mix of the NPIs based on the density and living conditions of the community.
COVID-19; Forecast; Islands; Non-pharmaceutical interventions; Modelling
COVID-19 has spread across 215 countries and territories worldwide with more than 31 million cases and almost one million deaths as of 18 September 2020 [1]. The rapidly spreading COVID-19 pandemic that emerged from Wuhan China in December 2019 was imported to the small islands of Maldives through travelers with the first case being reported on 7 March 2020 [2]. In the Maldives, COVID-19 was first detected in a tourist resort and in one week, 13 cases were confirmed with more than 300 people exposed to the cases [3] Therefore, the ability to accurately understand and predict the pandemic dynamics in the unique environment of islands is of critical importance in responding to the COVID-19 in Maldives.
Compartmental models [4] have been used to analyze numerous influenza studies in the past and other epidemiological outbreaks including the H1N1 pandemics of 1918 and the 1968-1971 H3N2. For the COVID-19 pandemic, these modelling approaches were used to predict pandemic dynamics [5] to forecast the spread [6] and to estimate mortality ratios [7]. There are also modelling studies developed for non-pharmaceutical interventions (NPIs) and COVID-19 [8], showing the effect of control measures, to assess the potential severity and transmissibility of the pandemic, and to determine the contagious period of this novel viral strain.
Unlike studies of pandemics that spread in huge population and landlocked countries, only a few limited studies have looked into its spread in island settings which can have natural containment dynamics. In 1971, Mathews JD, et al. [9], estimated R0 of an island population for H3N2 outbreak, where the median R0 of 6.4 (95% credibility interval 3.7-10.7). This meant that most of the people living in the small community were exposed twice, but exposure from the first wave provided protective immunity in the community and few became ill during the second wave. Yet the infection rate was high with an R0 =6.4, which indicates that the proportion of people who became immune from the first wave was low and reflects that transmission of the virus is high among small communities. The findings are similar to that in a closed setting, with a high infection rate posing a higher risk of community spread in islands.
The small islands in the Maldives, surrounded by sea make containment easy, yet it becomes a challenge to effectively isolate those on the island. However, due to the different population density in the capital island of Maldives and other islands, we modelled the effects of the NPIs on the capital and other islands separately. This is the first time the data is publicly available opening a unique opportunity to research the COVID-19 pandemic spreading through the geographically contained island communities.
The mathematical model aims to determine the epidemic dynamics of COVID-19, quantify the impact of the non-pharmaceutical interventions (NPIs) and forecast infection parameters.
To formulate and develop the values for each compartment we developed an optimal model using the following assumptions (Table 1).
List of notations | Description | Values |
s(t) | Susceptible population at time t (t=0,1,2…n) Note: average island population size | S(t0)=313,854 (Male’) S(t0)=1,837 (islands) [10] |
i(t) | Infected population at time t | I(t0)=2 |
r(t) | Recovered population at time t | R(t0)=0 |
d(t) | Deaths at time t | D(t0)=0 |
u(t) | Decision variable to model containment measure; u(t)=pxqxb | U(t0)=0 |
β | Infection rate; β=pxq | 5.74×10ˆ-6 for Male’ 4.78×10ˆ-6 for islands |
p | Probability of contact with infected person; p=k/N | |
P=12/313,854 (Male’)* | 3.82×10ˆ-5 for Male’ | |
P=10/1,837 (islands) | 5.44×10ˆ-3 for islands | |
q | Probability falling ill if contact with an infected person | 0.15 [11] |
k | Average number of adequate contacts made by an infected individual per time. *Note: The first 13 cases are used to calculate contacts per person which is12 for Male’ region and 10 for islands. Thus, these exact values are used to calculate infection rate. |
12 for Male’ 10 for islands |
N | Total population size (National Bureau of Statistics, 2020) with 100,000 undocumented immigrants. Assumed that 80% of migrants will reside in Male’ and 20% of migrants will be distributed equally among 187 islands). |
N(t)=313,854 (Male’) N(t)=1,837 (islands) [10] |
τ | Rate of death: approximated average mortality rate of the disease. | 0.02 [6,12,13] |
γ | Rate of recovery; γ=(1-τ) Everyone will recover except the percentage that dies. |
0.98 [13] |
b | Reduction in infection by implementation of containment measures | Refer to table 2 |
Table 1: List of notations used in the model.
a) This model comprised four compartments Susceptible as S(t), Infected as I(t), Recovered as R(t) and Deaths as D(t) at any time t ≥ 0. The population N(t) [14] is assumed to have natural containment due to island structures of the Maldives, without any demographic changes such as births or deaths due to natural causes, therefore at a point the population of Maldives can be described using:
N(t) =S(t)+ I(t) +R(t) +D(t) (1)
b) The people can contact each other and is homogeneous unless otherwise stated by the NPI. Where NPI is modelled by exposure variable u(t), where 0 ≤ u(t) ≤ b < β. Since most of the NPIs involves reducing contact within the population, once stringent measure is implemented the rate of infection is lowered from b at Do Nothing scenario to b-u(t) for all other scenarios.
c) Only people from the susceptible state can move to infected compartment. Once infected they can either die at a constant rate, τ or recover at a constant rate, g. It should be noted that the whole population is susceptible, and only from this state people get infected. This means the virus kills τ of the people it infects, the people who recover (1-τ) will move to recovered compartment.
Based on these assumptions, the following schematic diagram (Figure 1) can be used to depict the four compartments of SIRD model: Susceptible(S), Infected(I), Recovered(R), Deceased(D).
Data collection
The epidemiological data on COVID-19 was published by the Health Protection Agency (HPA), Maldives, daily from the start of the epidemic until the end of March 2020. The data for the forecast that was obtained from the HPA included information on number of people tested for COVID-19, number of contacts traced, number of those identified as suspects and the number quarantined or isolated.
The susceptible population for Male’ and an average island (small island) were derived from the population figures published by the National Bureau of Statistics [10]. For the Male’ population, a total of 313,854 is used which includes local residents and estimated foreign migrant population. Small islands are considered to have a population of 1,837 which is an average of the population of the rest of the 187 islands
In the model, the susceptible population (S) moves to infected compartment at the rate “β” if they come into contact with an infectious person, thus reducing the susceptible population. This controlled dynamics is used in similar NPI setting [14] and denoted by the time derivatives which are non-linear differential equations. Therefore, the time derivatives for the variables in the model are represented as follows:
ṡ(t)=−(β − u(t))s(t)i(t) (2)
İ(t)=(β − u(t))s(t)i(t)−(γ+τ)i(t) (3)
ṙ(t)= γi(t) (4)
ḋ(t)=τi(t) (5)
Where the sum of all-time derivatives is equal to 1, i.e.: ṡ(t)+İ(t)+ṙ(t)+ḋ(t)=1 s(t), i(t), r(t), d(t) ≥ 0, and the control, u(t). Thus, we calculated the number of people in each of the compartment S, I, R, D at t1, t2, t3 … tn.
Experiments
The SIRD model was applied for different rates of protection for each implemented containment measure applied in the islands. The effect of the NPIs were quantified and compared with the “Do Nothing” scenario, to investigate whether the spread of epidemic could have been prevented using NPIs, what proportion of the population was susceptible over the course of the epidemic, and how many new infections and deaths were generated by the NPIs. The outbreak was simulated using parameter values from the actual numbers on the first 13 cases in the Maldives and values from the literature, fitted to the geospatial and societal context of the Maldives (Table 2). Each simulation started with 2 infected individuals at t0 , with the rest of the population being in the susceptible state.
Scenarios | Definition | Effect value | Reviewed papers |
No NPI: Do nothing | All exposed | 0% | - |
NPI1: | Combination of Thermal screening, checking of travel history and health declaration form screening, case isolations and quarantine all suspects at a facility: no exposure to public or HH members (Quarantine and Isolation) | 18% | Anderson RM, et al., Browne C, et al., Keeling MJ, et al., Klinkenberg D, et al., Müller J, et al., Rivers CM, et al. [15-20] |
NPI2: | NPI1 and no mass gatherings, including social, public and religious gatherings (No mass gatherings) | 25% | Anderson RM, et al., Gastañaduy PA, et al., Kelso JK, et al., Pasquini-Descomps H, et al., Uscher-Pines L, et al. [15,21- 24] |
NPI3: | NPI1, NPI2 and Social distancing within government, institution-based businesses and education (Social distancing) | 32% | |
NPI4: | NPI1, NPI2, NPI3 and all social activities prohibited: Closure of school, universities, café's, gyms, spas, mosques, social visits, government and business closure (Restrictive social distancing). | 38% | (Ahmed F, et al., Ball F, et al., Chu CY, et al., Halloran ME, et al., Kleczkowski A, et al., Milne GJ, et al. [25- 30]). |
NPI5: | NPI1, NPI2, NPI3, NPI4 and closing of border, closing shops, businesses, island closures (Semi-lockdown). | 43.4% | (Bi Q, et al., Chung LH, et al., Selvey LA, et al., Tay J, et al., WHO [31-35]) |
Table 2: NPI categories, definitions and assumed reduction in infection by implementation of NPIs.
Ethical Issues/Statement
Ethical approval was sought from National Emergency Operations Committee (NEOC) established to oversee COVID-19 operations and National Health Research Council (NHRC) of Ministry of Health, Maldives.
The model output showed that stringent NPIs in combination can substantially reduce R0 , distilling the effect of the epidemic on the health system and reducing the deaths (Figure 2 and Figure 3). However, when the NPIs are implemented as a single intervention, the effect is less visible.
When the model is run with assumption that all the simulations are not-mutually exclusive, unless otherwise stated, with the b values fitted to Maldivian context, the total infections, total deaths, duration of the epidemic and R0 changed to various control measures. This model suggests that change in ‘b’ values affected by all the nonpharmaceutical interventions, delay the peak incidence, as well as the peak of the outbreak and lengthening the epidemic (Table 3).
Figure 2: Estimated infections in Male’.
Figure 3: Estimated deaths in Male’.
Male’ (Capital island) | Small Island in Maldives | |||||||
Total infections | Total deaths | Duration in days | R0 | Total infections |
Total deaths | Duration in days | R0 | |
No NPI | 248,616 | 4,972 | 35 | 1.8 | 1,139 | 23 | 30 | 1.5 |
-79.20% | -1.60% | -62.00% | -1.20% | |||||
NPI1 | 188,385 | 3,768 | 54 | 1.5 | 670 | 13 | 51 | 1.3 |
-60.00% | -1.20% | -36.50% | -0.70% | |||||
NPI2 | 154,176 | 3,084 | 69 | 1.4 | 414 | 8 | 72 | 1.1 |
-49.10% | -1.00% | -22.50% | -0.50% | |||||
NPI3 | 110,878 | 2,218 | 100 | 1.2 | 127 | 3 | 104 | 1 |
-35.30% | -0.70% | -6.90% | -0.10% | |||||
NPI4 | 64,045 | 1,281 | 170 | 1.1 | 26 | 1 | 37 | 0.9 |
-20.40% | -0.40% | -1.40% | 0.00% | |||||
NPI5 | 3,553 | 71 | 206 | 1 | 13 | 0 | 18 | 0.9 |
-1.10% | 0.00% | -0.70% | 0.00% |
Table 3: Infections, deaths, duration and change in reproduction number due to control measures.
For a small population island in the Maldives, without any social distancing scenarios, infections peak at 62% while deaths are at 1.2% of population. From NPI1 to NPI3 the duration of epidemic increases, however NPI4 (restrictive social distancing) and NPI5 (semilockdown) shows a short epidemic duration with a reproduction number less than 1 (Figure 4 and Figure 5).
Figure 4: Estimated infections in an island.
Figure 5: Estimated deaths in an island.
The unique geographic setting of Maldives restricts the population to small communities varying from 200 to 2000 people in most inhabited islands, except a few inhabited islands and the capital Male’, where more than a third of the population resides. By using the real epidemic data for Maldives, the model is fitted with nonlinear optimization, to estimate the transmission parameters in the Maldives islands, to Male’ and average sized small island scenarios. Unlike many early studies of COVID-19 transmission that considered either huge populations like China, its cities [36], Italy and the international spread of infection [14], the investigation of the pandemic, with assumed ‘b’ values for the effect of different NPIs taking into consideration the atoll/island setting of the Maldives islands with a SIRD model, provided the best fit given the high transmission and reported fatality of the disease.
The epidemic dynamics are different in Male’ compared to the average small island. The effect of containment measures appears to be more effective in the small island settings which is, likely to be driven by the smaller population and also the smaller number of exposures due to differences in housing characteristics in the two settings. This observation is in contrast to findings of Mathews and colleague [9] for island settings, but more likely in relation to the situation in Male’ Male’ which has a high population density and a number of communal living arrangements among the migrant labour force [37].
The results of the model appear to be realistic, considering the multiple but synergistic efforts by different stakeholders involved in the national emergency activities in creating awareness and infection prevention and control measures. The HPA issued daily reports on the pandemic, urging individuals to maintain hand hygiene in local and foreign languages, and indicating measures they could take to avoid infection. Furthermore, the population is well connected socially as well as digitally, and the young population structure in the country makes them high social media users [38].
Without any containment measures and any disruptions to the social or economic movements of the society (the “Do Nothing” scenario), high mortality and morbidity is predicted due to the COVID-19 transmission, in both Male’ (capital city) and in the small island settings. However, with NPI1 (where only quarantine and isolation of the cases are observed) and NPI2 (where in addition to NPI1, mass gatherings are restricted) mortality is reduced. In these two scenarios the epidemic duration is extended however the number of infections continues to be quite large and likely to cripple the health system. However, NPI3 (the same as NP2 with additional social distancing measures within the community and businesses), the effect is markedly different in both the small island setting and Male’. This is of the Male’. Low levels utmost important as it indicates minimal containment measure is effective for small islands, but less effective in of restrictions are encouraging as the living conditions in mall islands will not be severely affected and community can function at a state near to normal. This is observed in the current situation of Maldives, where the epidemic spread has been easily contained without taking measures of NPI4 and NPI5 in small islands outside Male’ [3]. However, the case for Male’ is different as it requires NPI4 (where all social activities are restricted) and NPI5 (semi-lockdown measures) to contain the epidemic, consistent with actual observed in the current epidemic situation in the Maldives. While the semilockdown halted the spread of the epidemic in Male’ it affected the livelihoods and social support mechanisms. The ease of lockdown to NPI3 level in Male’ resulted in surge of cases which required further restrictions as in NPI4 [3]. This is consistent with the model outputs that the control of the epidemic in Male’ can be achieved with some restrictions of movement and daily life, while movement restrictions need not be enforced in small islands.
Numerous mathematical modelling is emerging to examine the COVID-19 epidemic, although to the authors’ knowledge this is the first modelling study done for an island nation or small populations. While most simulations and modelling studies approach to predict the future pandemic dynamics, this model is applied for the current NPIs applied in the Maldives, modelling the effects of each NPI at a stage where community spread of COVID-19 starts in the small islands.
The results suggest that the more stringent NPIs which include a combination of social distancing measures give protection to the population. The susceptible compartment constitutes a large number of the population in each of the scenario, except the “Do Nothing” scenario, at which point, more new infections spread to this compartment and is converted to the infection compartment. The results are sensitive to the NPIs and identify the importance of the NPIs in containing the epidemic. The model indicates that in small islands, NPIs if combined and stringent enough would be sufficient to reduce R0 below 1, similar to scenario 3, which limits mass and public gatherings but allows government and institutional businesses and education to function.
The susceptible compartment increases in size with the NPIs implemented, which might be due to several overlapping interventions instituted prior to the reporting of the first case in the Maldives context that determined the probability of exposure. The NPIs implemented include screening at the ports of entry even before the COVID-19 was imported to Maldives, and all the arrivals to the country, irrespective from where they arrive, being held at quarantine and isolation facilities depending on their clinical status. In modelling the scenarios, the effects of these two NPIs are considered to be in place, with respect to the other NPIs. Therefore, the susceptible population is likely to have remained at high level.
Finding reliable transmission data in small island populations to shed light on COVID-19 dynamics is presently difficult, as was the case during the 2009 H1N1 pandemic [9] and are confounded by inconsistent data collection, results and emerging new findings. Thus, it is noted that R0 for countries differ, and is the same in Maldives with R0 between 1-2. Without comparable data from a small island population, basic transmission parameters, including R0, can be more accurately determined for a smaller island state like Maldives.
This model is limited to a population of a small island states, geographically dispersed and may not be generalizable to land-locked countries. However, the results are relevant for many settings that share characteristics of Maldives-namely, small islands which are separated by sea with low but close-knit populations. Secondly, this model assumed a closed population in which the small population did not leave for the duration of the pandemic, given the current movement control restriction applied. However, in the medium term, it is unlikely to be a strictly closed population as a small percentage of population may travel in and out of the community. Thirdly, currently available transmission data for the novel virus is used here, however, with new research this analysis may need to be repeated with the new data. There is also a need to further explore the effects of the measures during the community spread and expand modelling to plan resources for the community spread scenarios to reduce the health system burden.
The model applied to Maldives provides a deeper insight into the dynamics of the spread of COVID-19 and quantify the impact of the NPIs in a small island context. The effect of implementation of multiple NPIs before and early at the onset of the community spread is seen in reducing R0 below 1.0 and protects a large portion of the population. The model produces different outputs for the measures in the small island setting compared to the more densely populated capital city island which is consistent with what is currently observed in Maldives. While the epidemic can be controlled via quarantine and isolation along with social distancing practices without much restriction on day-to-day life in the less congested small island context, more restrictions on work and daily activities have to be applied in Male’ to control the spread. The model provides valuable information for containment planning for small island settings (similar to a closed setting), which have limited resources to respond to community spread. Hence, the findings may not be generalized to large and open populations. Further modelling with actual data from the community spread of the epidemic is needed to refine the model assumptions that can more accurately guide response in small island developing country settings.
All authors have read and given input to the manuscript.
This work is done out of academic interest.
None
None
We thank Dr. Aishath Shehenaz Adam, Vice Chancellor for Research at the Maldives National University for her support.
- Johns Hopkins University (2020) Coronavirus COVID-19 Global Cases by the Center for Systems Science and Engineering (CSSE) at Johns Hopkins University (JHU). [Ref.]
- World Health Organisation (2020a) Coronavirus disease 2019 (COVID-19): Situation Reports. WHO, Geneva, Switzerland. [Ref.]
- Ministry of Health, Republic of Maldives (2020) COVID-19 Local Updates. [Ref.]
- Brauer F (2008) Compartmental Models in Epidemiology. In: Brauer, F, van den Driessche, Wu J (eds) Mathematical Epidemiology. Springer-Veralg Berlin Heidelberg 19-79. [Ref.]
- Fang Y, Nie Y, Penny M (2020) Transmission dynamics of the COVID-19 outbreak and effectiveness of government interventions: A data-driven analysis. J Med Virol 92: 645-659. [Ref.]
- Wu JT, Leung K, Bushman M, Kishore N, Niehus R, et al. (2020) Estimating clinical severity of COVID-19 from the transmission dynamics in Wuhan, China. Nat Med 26: 506-510. [Ref.]
- Hauser A, Counotte MJ, Margossian CC, Konstantinoudis G, Low N, et al. (2020) Estimation of SARS-CoV-2 mortality during the early stages of an epidemic: A modeling study in Hubei, China, and six regions in Europe. PLoS Med 17: e1003189. [Ref.]
- Ferguson NM, Daniel L, Nedjati-Gilani G, Imai N, Ainslie K, et al. (2020) Report 9: Impact of non-pharmaceutical interventions (NPIs) to reduce COVID-19 mortality and healthcare demand. Imperial College London, United Kingdom. [Ref.]
- Mathews JD, McCaw CT, McVernon J, McBryde ES, McCaw JM (2007) A biological model for influenza transmission: pandemic planning implications of asymptomatic infection and immunity. PLoS ONE 2: e1220. [Ref.]
- National Bureau of Statistics, Ministry of Finance and Treasury (2020) Maldives Population Projections 2014-2054. [Ref.]
- Yang P, Ding Y, Xu Z, Pu R, Li P, et al. (2020) Epidemiological and clinical features of COVID-19 patients with and without pneumonia in Beijing, China. medRxiv 1-31. [Ref.]
- Verity R, Okell LC, Dorigatti I, Winskill P, Whittaker C, et al. (2020) Estimates of the severity of coronavirus disease 2019: a modelbased analysis. Lancet Infect Dis 20: 669-677. [Ref.]
- Wu Z, McGoogan JM (2020) Characteristics of and Important Lessons from the Coronavirus Disease 2019 (COVID-19) Outbreak in China: Summary of a Report of 72 314 Cases from the Chinese Center for Disease Control and Prevention. JAMA 323: 1239-1242. [Ref.]
- Lin F, Muthuraman K, Lawley M (2010) An optimal control theory approach to non-pharmaceutical interventions. BMC Infect Dis 10: 32. [Ref.]
- Anderson RM, Heesterbeek H, Klinkenberg D, Hollingsworth TD (2020) How will country-based mitigation measures influence the course of the COVID-19 epidemic? Lancet 395: 931-934. [Ref.]
- Browne C, Gulbudak H, Webb G (2015) Modeling contact tracing in outbreaks with application to Ebola. J Theor Biol 384: 33-49. [Ref.]
- Keeling MJ, Hollingsworth TD, Read JM (2020) Efficacy of contact tracing for the containment of the 2019 novel coronavirus (COVID-19). J Epidemiol Community Health 74: 861-866. [Ref.]
- Klinkenberg D, Fraser C, Heesterbeek H (2006) The effectiveness of contact tracing in emerging epidemics. PLoS ONE 1: e12. [Ref.]
- Müller J, Kretzschmar M, Dietz K (2000) Contact tracing in stochastic and deterministic epidemic models. Math Biosci 164:39-64. [Ref.]
- Rivers CM, Lofgren ET, Marathe M, Eubank S, Lewis BL (2014) Modeling the impact of interventions on an epidemic of ebola in sierra leone and liberia. PLoS Curr 6. [Ref.]
- Gastañaduy PA, Funk S, Paul P, Tatham L, Fisher N, et al. (2018) Impact of Public Health Responses during a Measles Outbreak in an Amish Community in Ohio: Modeling the Dynamics of Transmission. Am J Epidemiol 187: 2002-2010. [Ref.]
- Kelso JK, Milne GJ, Kelly H (2009) Simulation suggests that rapid activation of social distancing can arrest epidemic development due to a novel strain of influenza. BMC Public Health 9: 117. [Ref.]
- Pasquini-Descomps H, Brender N, Maradan D (2017) Value for Money in H1N1 Influenza: A Systematic Review of the Cost- Effectiveness of Pandemic Interventions. Value Health 20: 819-827. [Ref.]
- Uscher-Pines L, Schwartz HL, Ahmed F, Zheteyeva Y, Meza E, et al. (2018) School practices to promote social distancing in K-12 schools: review of influenza pandemic policies and practices. BMC Public Health 18: 406. [Ref.]
- Ahmed F, Zviedrite N, Uzicanin A (2018) Effectiveness of workplace social distancing measures in reducing influenza transmission: a systematic review. BMC Public Health 18: 518. [Ref.]
- Ball F, Britton T, Leung KY, Sirl D (2019) A stochastic SIR network epidemic model with preventive dropping of edges. J Math Biol 78: 1875-1951. [Ref.]
- Chu CY, de Silva UC, Guo J-P, Wang Y, Wen L, et al. (2017) Combined interventions for mitigation of an influenza A (H1N1) 2009 outbreak in a physical training camp in Beijing, China. Int J Infect Dis 60: 77-82. [Ref.]
- Halloran ME, Ferguson NM, Eubank S, Longini IM, Cummings DAT, et al. (2008). Modeling targeted layered containment of an influenza pandemic in the United States. Proc Natl Acad Sci U S A 105: 4639- 4644. [Ref.]
- Kleczkowski A, Maharaj S, Rasmussen S, Williams L, Cairns N (2015) Spontaneous social distancing in response to a simulated epidemic: a virtual experiment. BMC Public Health 15: 1-13. [Ref.]
- Milne GJ, Baskaran P, Halder N, Karl S, Kelso J (2013) Pandemic influenza in Papua New Guinea: a modelling study comparison with pandemic spread in a developed country. BMJ Open 3: e002518. [Ref.]
- Bi Q, Wu Y, Mei S, Ye C, Zou X, et al. (2020) Epidemiology and transmission of COVID-19 in 391 cases and 1286 of their close contacts in Shenzhen, China: a retrospective cohort study. Lancet Infect Dis 20: 911-919. [Ref.]
- Chung LH (2015) Impact of pandemic control over airport economics: Reconciling public health with airport business through a streamlined approach in pandemic control. J Air Transp Manag 44: 42-53. [Ref.]
- Selvey LA, Antão C, Hall R (2015) Evaluation of Border Entry Screening for Infectious Diseases in Humans. Emerg Infect Dis 21: 197-201. [Ref.]
- Tay J, Ng YF, Cutter JL, James L (2010) Influenza A (H1N1- 2009) pandemic in Singapore--public health control measures implemented and lessons learnt. Ann Acad Med Singap 39: 313-412. [Ref.]
- World Health Organisation (2020b) Global surveillance for COVID-19 caused by human infection with COVID-19 virus. Interim guidance, WHO, Geneva, Switzerland. [Ref.]
- Xia W, Sanyi T, Yong C, Xiaomei F, Yanni X, et al. (2020) When will be the resumption of work in Wuhan and its surrounding areas during COVID-19 epidemic? A data-driven network modeling analysis. Sci Sin Math 50: 1674-7216. [Ref.]
- International Organization for Migration (2020) Maldives Migration Profile Highlights Key Role of Foreign Migrant Workers. IOM, Switzerland. [Ref.]
- Ministry of Health, ICF (2018) Maldives Demographic and Health Survey 2016-2017. Republic of Maldives. [Ref.]
Download Provisional PDF Here
Article Type: RESEARCH ARTICLE
Citation: Usman SK, Suzana M, Moosa S (2020) Differential Forecasting of the Spread of COVID-19 in Small Islands with Containment Interventions. J Epidemiol Public Health Rev 5(4): dx.doi.org/10.16966/2471-8211.201
Copyright: © 2020 Usman SK, et al. This is an open-access article distributed under the terms of the Creative Commons Attribution License, which permits unrestricted use, distribution, and reproduction in any medium, provided the original author and source are credited.
Publication history:
SCI FORSCHEN JOURNALS
All Sci Forschen Journals are Open Access