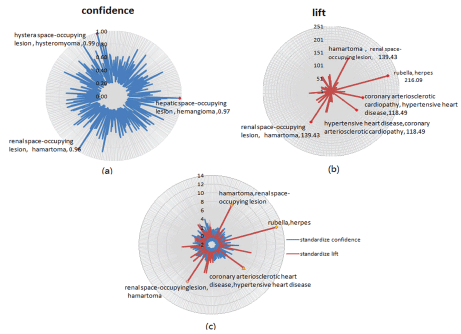
Figure 1: Radar charts of (a) confidence, (b) lift and (c) standardized confidence and lift values for association rules among diseases and physical signs.
Hua Chen1# Shan Hu2# Zhixiao Luo3# Lan Tang3 Qing Zeng1 Xiaoyan Wen1 Jiangpeng Chen1 Ping Que1 Bin Peng11*#
1School of Public Health and Management, Chongqing Medical University; Research Center for Medicine and Social Development; Innovation Center for Social Risk Governance in Health, Chongqing, China*Corresponding author: Bin Peng, School of Public Health and Management, Chongqing Medical University, Chongqing, China, E-mail: pengbin@cqmu.edu.cn
Association rule mining has been well researched as a data mining method for discovering new and interesting knowledge in large databases. In this study, association rule mining technique was used to discover human disease-disease associations from a physical examination dataset. These disease-disease associations were visualized by networks, which have become a holistic approach used to understand complex relationships among diseases. The results showed that 47 percent of 247073 individuals suffered from dyslipidemia, while 24 percent suffered from fatty liver. Moreover, dyslipidemia was highly related to various diseases and physical signs, such as obstructive sleep apnea syndrome (confidence=0.83, lift=1.76), unusually high glutamyltransferase (confidence=0.82, lift=1.73) and hyperviscosity (confidence=0.81, lift=1.72). The strongest rule discovered in this study was (hystera space-occupying lesions ⇒ hysteromyoma) with a confidence of 0.99 and a lift of 18.99. Meanwhile, some novel relationships were extracted. For example, an enlarged gallbladder closely related to prostatic hyperplasia. This rule had a confidence of 0.78 and a lift of 41.49, which means among the patients with enlarged gallbladders, 78 percent also suffered from prostatic hyperplasia. This paper discovered many novel associations, some of which were rarely reported and even if reported in previous studies, but the perspectives and results were still not consistent. Therefore, disease association networks are valuable for clinicians and medical researchers to examine the relationships among diseases and physical signs.
Disease association; Disease network; Association rule mining; Physical examination database
A number of studies have revealed that human diseases form an interconnected landscape [1-4]. A pair of diseases, even those affecting different organs or with distinct symptoms, can be related due to dysfunction of the same genes or their disease-associated proteins may act in the same pathway [1,3,5]. The disease associations have important implications for disease prevention, diagnosis, and treatment, which also can be helpful to related researches. Therefore, more and more researchers have been studying disease associations via medicine databases that touch on the concepts of disease connections, such as Genetic Association Database (GAD) [6] and Online Mendelian Inheritance in Man (OMIM) [7], which produce disease-disease associations by constructing genegene associations or protein-protein associations [7]. However, these introduced some biases. First, few of these databases utilized both comprehensive omics and biological literature corpus to predict diseasedisease relationships [5]. Although some studies have considered the data obtained from literature to detect a disease-gene association, they only included a few diseases rather than overall diseases [8-10]. Second, it is evident that obtaining a catalog of the disease-related genes is not sufficient for those complex diseases such as cardiovascular disease, type 2 diabetes, cancer because phenotypic outcomes cannot be predicted solely based on genotypes [11,12]. For example, alterations in several genes can make subtle contributions to the susceptibility of a particular individual, which makes it difficult to select the candidate genes or single nucleotide polymorphisms (SNP) [13]. Therefore, it is necessary and of great importance to find new breakthrough points and new methods to study disease-disease associations. Association rule mining has been developed to extract high-quality relevant information [14-18]. In this paper, it was creatively used to discover disease associations from varieties of diagnosis based on large physical examination database.
Currently, physical examination holds a high proportion of health management in China [19]. It can say that health management industry basically just contained physical examination in many cities. As a disease prevention measure of early detection, diagnosis, and treatment, a physical examination is becoming more and more popular [20]. To optimize the process of physical examination and to improve the efficiency of medical management, physical examination management system has been widely used in hospitals and health management companies. With the increasing amount of physical examination data, it’s a very meaningful task to find valuable information or knowledge, especially in the era of big data [21]. However, the current studies of physical examination databases were limited to a few diseases, such as dyslipidemia, fatty liver disease [22,23]. Therefore, it is important to do more diverse and global research on disease associations, which can discover more amusing and innovative information from physical examination databases.
The data came from the First Affiliated Hospital of Chongqing Medical University, which was the data protection agency. The patients’ physical examination records were anonymized before being accessed for analysis. And this study was approved by the research ethics committee of Chongqing Medical University.
In this study, a total of 247,073 medical examination records were included, which were reported by the First Affiliated Hospital of Chongqing Medical University. The information involved sex, age, blood glucose, blood pressure, radiation test results, and medical diagnosis. The medical diagnosis, containing diseases and physical signs, can reflect different health problems of individuals, from which the disease-disease associations were extracted.
An association rule, denoted by A ⇒ B, was used to describe events that tend to occur together or to reflect the interdependence and correlation between one event and other events [24]. Here A or B was a set of events. Therefore, if there are associations among two or more events, we can predict one through the others. Given a set of medical diagnosis, the problem of mining association rules is to generate rules that have a support and a confidence greater than or equal to a userspecified minimum support and confidence levels; these rules are called strong association rules [25]. The support of an event, i.e. disease, is the proportion of medical records in the dataset that contain this event, which measures how frequently an event occurs. The confidence of a rule A ⇒ B is the conditional probability of B given A. Alternatively it’s a statement about the occurrence of B in A, which measures how confidently a rule is. Another popular measure for an association rule is lifted, which can be interpreted as the deviation of the support of a rule from the support expected under independence given the support of both sides of the rule. In other words, lift (A ⇒ B)=confidence(A ⇒ B)/P(B). A lift around 1 means that the occurrence of A and the occurrence of B in the same transaction are independent events, therefore, A and B were not related. It is easy to show that the lift is greater than 1 indicates that the strength of the correlation between event set A and set B [26,27].
In this study, the Apriori algorithm was used to discover overall disease-disease associations. The core idea of Apriori algorithm is to make multiple passes over the database. It uses an iterative method called breadth-first search through the search space, in which k-itemsets are used to explore (k+1)-itemset. It identifies frequent single items in the database and extends them to larger and larger itemsets, as long as these items often appear in the database [28]. Various algorithms have been proposed for mining association rule such as AIS algorithm, Apriori algorithm, Apriori-tid algorithm, Apriori-Hybrid, etc. but in every algorithm there founds a common drawback of various scans over the database and the Apriori algorithm is the most widely used and most classic association rule algorithm [29]. In order to mine frequent and strong association rules, the minimum support and minimum confidence values were set as 0.001 and 0.2, respectively. To apply an association rule algorithm, R-Studio 7.2 was used.
These network-based approaches provide many nice visual models to study relationships among objects in terms of nodes and links [30-32]. In order to understand various disease associations better, disease networks were constructed according to discovered association rules. In these networks, a node represents a disease or a physical sign; a link represents a relationship between two nodes. They enable us to globally understand the relationships among diseases and physical signs. A disease network can be characterized by three basic invariant quantities: degree, average path length, and clustering coefficient, which are measurements related to the connectivity, size and density of the network, respectively [33,34]. The disease networks were visualized using Cytoscape, which is an open source software platform for visualizing complex networks [35].
Among the 247,073 individuals who had one or more diseases or physical signs, 47 percent suffered from dyslipidemia, 24 percent suffered from fatty liver disease and 23 percent were overweight. The top 20 frequent diseases and physical signs were presented in Table 1.
Disease | Frequency | Percent (%) |
dyslipidemia | 116310 | 47.08 |
fatty liver | 59883 | 24.24 |
overweight | 57204 | 23.15 |
hyperuricemia | 37601 | 15.22 |
breast hyperplasia | 37004 | 14.98 |
pulmonary grain | 34650 | 14.02 |
hypertension | 29308 | 11.86 |
ametropia | 25960 | 10.51 |
abnormal urine | 24458 | 9.9 |
hemorrhoids | 22528 | 9.12 |
cervical erosion | 22485 | 9.1 |
naboth cyst | 20326 | 8.23 |
hepatic cyst | 19969 | 8.08 |
High blood pressure | 19829 | 8.03 |
hepatic dysfunction | 18228 | 7.38 |
cataract | 15761 | 6.38 |
renal cyst | 15176 | 6.14 |
diabetes | 12958 | 5.24 |
hysteromyoma | 12940 | 5.24 |
Table 1: The top 20 frequent diseases.
From 247,073 pieces of records, 143 frequent diseases or physical signs and 628 rules were extracted. Here in Table 2 we listed the 23 association rules with a confidence value of at least 0.7 and a lift value of at least 1.5. As mentioned previously, the two numbers were used to measure the strength of a rule. For example, the rule (hystera space-occupying lesion ⇒ hysteromyoma) implied that hystera space-occupying lesion had a close relationship to hysteromyoma with a confidence value 0.99 and a lift value 18; likewise, the rule (hepatic space-occupying lesion ⇒ hepatic hemangioma) implied that hepatic space-occupying lesion closely related to hepatic hemangioma with a confidence value 0.97 and a lift value 49. The two rules suggested that hysteromyoma and hepatic hemangioma were very likely to occur in those suffering from hystera space-occupying lesion. We also found that dyslipidemia was the consequent set of most association rules, as shown in Table 2. It may imply that dyslipidemia was highly related to a variety of diseases and physical signs and played an important role among disease associations.
Antecedent | Consequent | support | confidence | lift |
hystera space-occupying lesion | hysteromyoma | 0.039 | 0.99 | 19.00 |
hepatic space-occupying lesion | hemangioma | 0.014 | 0.97 | 49.81 |
renal space-occupying lesion | hamartoma | 0.005 | 0.96 | 139.43 |
aortic valve degeneration | Cardiomyopathy diastolic | 0.002 | 0.89 | 63.88 |
aortic valve degeneration | ventricle diastole function | 0.002 | 0.89 | 63.80 |
breast space-occupying lesion | Breast swelling | 0.007 | 0.87 | 82.05 |
obstructive sleep apnea syndrome | dyslipidemia | 0.007 | 0.83 | 1.76 |
unusually high glutamyltransferase | dyslipidemia | 0.022 | 0.82 | 1.73 |
eyeground arteriosclerosis | cataract | 0.013 | 0.81 | 12.71 |
hyperviscosity | dyslipidemia | 0.004 | 0.81 | 1.72 |
obesity | fatty liver | 0.020 | 0.80 | 3.32 |
hamartoma | renal space-occupying lesion | 0.010 | 0.79 | 139.43 |
left atrial enlargement | cardiomyopathy diastolic | 0.001 | 0.79 | 56.61 |
enlarged gallbladder | prostatic hyperplasia | 0.004 | 0.78 | 41.49 |
high myopia | ametropia | 0.038 | 0.78 | 7.39 |
hysteromyoma | hystera space-occupying lesion | 0.040 | 0.74 | 19.00 |
fatty liver | dyslipidemia | 0.180 | 0.74 | 1.58 |
coronary arteriosclerotic heart disease | hypertension | 0.002 | 0.73 | 6.16 |
high ferroprotein | dyslipidemia | 0.005 | 0.73 | 1.55 |
basal arteries blood flow decreased | dyslipidemia | 0.002 | 0.73 | 1.54 |
obesity | dyslipidemia | 0.018 | 0.72 | 1.53 |
frontal enlargement | pulmonary grain | 0.012 | 0.71 | 5.04 |
hemangioma | hepatic space-occupying lesion | 0.014 | 0.70 | 49.81 |
Table 2: Association rules of diseases (Confidence>0.7, Lift>1.5).
Only a small portion of the discovered association rules were shown in Table 2. In order to explore the strength of these rules, Radar Chart was used to visualize the confidence and lift values of each rule (Figure 1,1(a)) showed that most confidence values of the association rules were approximately between 0.2 and 0.6, and (Figure 1(b)) showed that most lift values were approximately from 1 to 51. (Figure 1(c)) showed the standardized confidence values and the standardized lift values simultaneously. A standardized value is the value minus the mean of all values over their standard deviation. As we can see, the following four rules have larger standardized lift values compared to their standardized confidence values: rubella ⇒ herpes, coronary arteriosclerotic heart disease ⇒ hypertensive heart disease, renal space-occupying lesion ⇒ hamartoma and hamartoma ⇒ renal space-occupying lesion. It implies that one right side disease of a rule appeared particularly in those suffering from the left side disease [26]. For example, herpes is particularly associated with rubella, and hypertensive heart disease is particularly associated with coronary arteriosclerotic heart disease.
Figure 1: Radar charts of (a) confidence, (b) lift and (c) standardized confidence and lift values for association rules among diseases and physical signs.
Disease network from association rules: The disease network was constructed according to the 628 discovered association rules (Figure 2). The right side diseases and left side diseases of an association rule are regarded as nodes and their association is represented by a link in Cytospace. The color of a node is determined by its degree, which is the number of links connected to other nodes. A node with higher degree is shown in deeper color, which implied a potentially more important hub in the disease network. The five red nodes with highest degree are dyslipidemia, fatty liver, overweight, hypertension and pulmonary grain shown in Figure 2. Additionally, the essential diseases and physical signs tended to be located at the functional center of the networks. Some physical signs played an indispensable role in the network, such as dyslipidemia, overweight and pulmonary grain.
Figure 2: Disease- Physical Sign Network from all association rules. Nodes represent diseases or physical signs; links represent associations among disease and physical signs.
The structural properties of a network can be measured by its average path length and clustering coefficient. Average path length describes the average number of steps along the shortest paths for all possible pairs of network nodes. Clustering coefficient, ranging from 0 to 1, usually used to measure the degree to which the nodes in a network tend to cluster together; value of 1 means every node is connected to every other node [36]. The average path length of our network is 2.008 and the clustering coefficient is 0.613. The average path length of 2.008 means the distance from one disease to any other diseases of network is only two steps. Therefore, we can conclude that the diseases and physical signs in the network are closely linked. Moreover, the position of a node in network mostly is decided by its degree. The node dyslipidemia had the highest degree of 141, followed by overweight and fatty liver disease with the same degree of 85. It means that dyslipidemia is related to 141 diseases or physical signs; overweight and fatty liver disease has association with 85 diseases or physical signs.
In order to highlight the strong associations among diseases and physical signs, the association rules with confidence values greater than 0.6 were extracted and shown in Figure 3. From this picture, we can easily find several diseases and physical signs are linked to dyslipidemia with a high confidence value around 0.6 to 0.85. Moreover, breast diseases (breast hyperplasia, fibroadenoma of breast, and breast space-occupying lesion) are highly related with each other, as shown in the lower left corner of Figure 3.
Figure 3: Disease-Physical Sign Network from association rules with confidence>0.6 Edge labels are the confidence of association rules. Higher values indicate the stronger associations.
A sub-network focused on a certain disease, which can be constructed via all of the association rules involving the disease. Through the subnetwork of a disease, one could understand better about the associations among its linked diseases. We picked out three diseases, i.e. hypertensive heart disease, arteriosclerosis and abnormal renal function, and extracted the corresponding association rules to construct the sub-network, respectively (Figure 4). The three diseases were limited in the left side sets of the association rules. Hypertensive heart disease is closely associated with dyslipidemia with a confidence of 0.59; hypertensive heart disease is also closely associated with arteriosclerosis and overweight with confidence values of 0.56 and 0.51, respectively. Therefore, among the patients with hypertensive heart disease, 59 percent had dyslipidemia, 56 percent suffered from arteriosclerosis and 51 percent are overweight (Figure 4(a)). Many diseases are highly related to arteriosclerosis with high confidence, such as cataracts, fatty liver disease, dyslipidemia, pulmonary grain and hypertension. Among these diseases, we should pay attention to the relationship between pulmonary grain and arteriosclerosis, which has not been paid enough attention to (Figure 4(b)). Additionally, the associations of abnormal renal function with other diseases or physical signs have been reported rarely. This study found seven diseases and physical signs are associated with abnormal renal function, including dyslipidemia, fatty liver disease, hypertension, overweight, pulmonary grain, renal cyst and arteriosclerosis (Figure 4(c)).
Figure 4: Sub-Networks of (a) hypertensive heart disease, (b) arteriosclerosis and (c) abnormal renal function.
It is worth noting that the above three selected diseases appeared on the three networks at the same time with high confidence. This pattern reflects their central positions in the three disease networks. Moreover, arteriosclerosis is also active in these disease networks; it has a close relationship with hypertensive heart disease and abnormal renal function.
In our study, we used an emerging data mining approach, called association rule mining, to construct disease networks so as to understand disease associations further. Compared with similar articles [37,38], we combined data mining with network, can discover the unknown, potential association among diseases. It offers insights that can potentially help us to understand high-risk diseases and progression pattern between recurrences. But, association rule mining could not determine any temporal or causal relationship. In the future research, we should try to combine association rules with epidemiological research methods.
Significantly, we discovered several disease associations, some of which have been well established, while others have not yet been confirmed, For example, our results revealed that 85 diseases and physical signs were associated with fatty liver disease. Among those associations, the relationships between hypertension, overweight, dyslipidemia and fatty liver disease were identified, which were already validated by previous studies and approved by domestic and overseas specialists [39-41]. Furthermore, we found that fatty liver disease has relationships with splenomegaly, hyperplasia of the prostate, abnormal renal function and kidney calculi. The relationship of fatty liver disease with abnormal renal function and kidney calculi was studied by Einollahi B et al. [42]. Their results showed that fatty liver disease may be a risk factor in the formations of calculi and is negatively associated with kidney function.
In our study, we extracted totally 628 association rules. However, many associations that were discovered among the diseases have not been paid enough attention to, such as splenomegaly, which was associated with fatty liver disease and prostatic hyperplasia with enlarged gallbladder. Moreover, this study contained disease-disease associations, disease-physical sign associations and physical sign-physical sign associations. Many researchers have ignored physical signs when studying disease associations. The idea of using physical signs to thoroughly understand disease associations may lead to further improvements in disease diagnosis, prognosis and treatment.
Association rule mining method and a graphical network were used to reveal the patterns of associations among diseases and physical signs. Association rule mining is an effective way to discover implicit information from complex disease associations. We used support, confidence and lift to assess the strength of these rules. In particular, the associations extracted by association rule mining were directive. Each disease can be an antecedent and/or a consequent. For example, there are two rules reflecting the relationship of cataracts and arteriosclerosis: cataract ⇒ arteriosclerosis, which have a confidence of 0.32 and a lift of 7.57, and arteriosclerosis ⇒ cataract, which have a confidence of 0.48 and a lift of 7.57. The two rules both have a high confidence, which suggests a close relationship between arteriosclerosis and cataracts; this relationship tends to be more reliable than one rule with a high confidence value. Moreover, due to the large sample size of this study, the association rules can be more reliable, and the networks generated by the association rules can provide significant characteristics of disease associations. However, it is noteworthy that association rule mining only describes the relationships between diseases that tend to occur together; it cannot explicitly reflect a cause-and-effect relationship. Therefore, we must further study the interesting associations combined with experiments, surveys or molecular biology tools.
This work was supported by grants from National Natural Science Foundation of China (Grant No. 81373103) and Chongqing Science & Technology Commission (Grant No. cstc2013jcyjA10009). The physical examination data for use in this study were supplied by the physical examination center of Chongqing Medical University. All of the analyses, interpretations and conclusions based on these data are solely that of the authors.
The authors declare that they have no competing interests.
Download Provisional PDF Here
Article Type: Research Article
Citation: Chen H, Hu S, Luo Z, Tang L, Zeng Q, et al. (2017) Study of Disease Networks Based on Association Rule Mining from Physical Examination Database. J Epidemiol Public Health Rev 2(6): doi http://dx.doi.org/10.16966/2471-8211.157
Copyright: © 2017 Chen H, et al. This is an open-access article distributed under the terms of the Creative Commons Attribution License, which permits unrestricted use, distribution, and reproduction in any medium, provided the original author and source are credited.
Publication history:
All Sci Forschen Journals are Open Access