Figure 1: Identification and inclusion process of the studies for the meta-analysis. 10 articles were included in our meta-analysis.
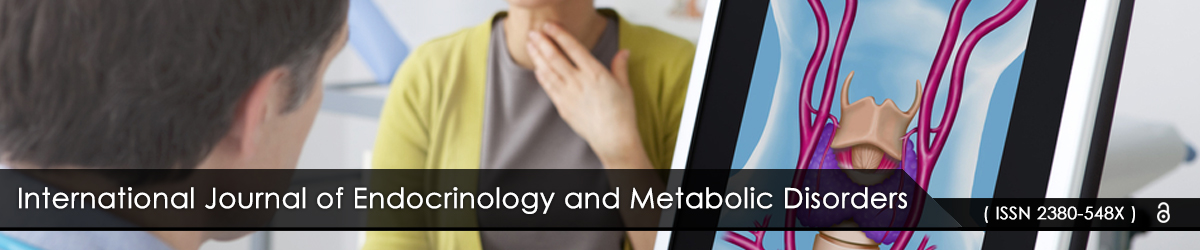
Full Text
Wei Li1-3,# Jia Zhou4,# Mei Yang5 Mengyao Deng5 Zhao Tang5 Yan Guo6*
1Department of Pharmacy, Maternal and Child Health Hospital of Hubei Province, Wuhan, China2Women and Children’s Hospital of Hubei Province, Wuhan, China
3Maternal and Child Health Hospital of Hubei Province, Tongji Medical College, Huazhong University of Science and Technology, Wuhan, China
4Yubei Maternal and Child Health Hospital of Chongqing Municipality, Chongqing, China
5Research Center for Health Promotion in Women, Youth and Children, Department of Maternal, Child and Adolescent Health, School of Medicine, Wuhan University of Science and Technology, Wuhan, China
6Wuhan Center for Disease Prevention and Control, Wuhan, China
#These authors contributed equally to this work
*Corresponding authors: Dr. Yan Guo, Wuhan Center for Disease Prevention and Control, Wuhan, China, No. 288 Machang Road, Jianghan District, Wuhan, Hubei 430021, PR China, China, E-Mail: guoyan8101@foxmail.com
Background: There were many studies detecting the relationship between Gestational Diabetes Mellitus (GDM) and Preterm Birth (PTB). However, the conclusions were inconsistent. Therefore, to clarify the relationship between GDM and PTB, a meta-analysis was conducted in this study.
Methods: Three computerized literature databases, PubMed, Web of Science and Cochrane Library, were systematically reviewed. The information and data from relevant studies based on incorporation criteria were selected and extracted. The pooled Odds Ratio (OR) with 95% Confidence Interval (CI) was calculated. Subgroup analyses were carried out according to geographical areas and GDM diagnostic criteria.
Results: A total of 10 studies met the inclusion criteria. Pooled results showed that GDM during pregnancy was a risk factor of PTB (OR=1.43, 95% CI 1.30-1.58), which was supported by subgroup analyses as well.
Conclusions: Women with GDM had an increased risk of PTB compared with those who had a normoglycemic pregnancy. Since the potential confounders could not be ruled out completely, further studies are needed to confirm these results.
Gestational diabetes mellitus; Preterm birth; Meta-analysis
GDM: Gestational Diabetes Mellitus; PTB: Preterm Birth; PRISMA: Preferred Reporting Items for Systematic Reviews and MetaAnalyses; MeSH: Medical Subject Headings; IADPSG: International Association of the Diabetes and Pregnancy Study Groups; NDDG: National Diabetes Data Group; DPSG: Diabetic Pregnancy Study Group; ICD: International Classification of Diseases; OR: Odds Ratio; CI: Confidence Interval
As one of the most common complications during pregnancy, gestational diabetes mellitus (GDM) is defined as carbohydrate intolerance of variable severity with onset or first recognition during pregnancy [1]. The incidence of GDM varies with geographic spreading. Approximately 2%-6% pregnancies have GDM in Europe [2], and the number of pregnant women affected by GDM in the United States has reached 9.2 % [3]. Moreover, the incidence of GDM in China is as high as 17.5% [4].
GDM increases the risk of adverse complications for pregnant women and its offspring, such as type 2 diabetes mellitus, shoulder dystocia, macrosomia and so on [5]. In addition, several studies also show that GDM might have a relationship with Preterm Birth (PTB). PTB is defined as the birth of an infant prior to 37 completed weeks of gestation [6]. As is one of the major causes that lead to perinatal morbidity and mortality [7], especially in middle-income and higher-income countries, PTB is accounting for 35% of the 3.1 million deaths worldwide each year [8]. In addition, PTB has lifelong effects on the neurodevelopmental function of surviving infants [9]. Therefore, it is necessary to understand the relationship between GDM and PTB, which may help to reduce the occurrence of PTB at the source.
Recently, several literatures showed that GDM was associated with PTB, but in other published data, it was concluded that there was no clear link between the two [10,11]. A compelling rationale basis based on evidence medicine to prove the relationship between GDM and PTB was scarce. Hence, the objective of this current study was to further confirm the relationship between GDM and PTB by evidencebased medical analysis.
Literature searching and screening were conducted according to the Preferred Reporting Items for Systematic Reviews and Meta-Analyses (PRISMA) guidelines [12].
Literature search
An electronic search of PubMed, Web of Science and Cochrane Library from inception to March 30, 2018, was conducted by two investigators independently (Jia Zhou and Mengyao Deng). The Medical Subject Headings (MeSH) and various synonyms were used to search the relevant articles. We used the following search terms: (premature birth) OR (premature labor) OR (preterm birth) OR “Premature Birth” [MeSh]) and (GDM) OR (gestational diabetes) OR (gestational diabetes mellitus, GDM) OR (diabetes in pregnancy) OR (gestational diabetes, GDM) OR (“Diabetes, Gestational” [MeSh]). Additional studies were identified by a manual search of the references of the original studies.
Criteria for inclusion and exclusion
This review followed these inclusion criteria: case-control studies or cohort studies; evaluating the effect of GDM on PTB; focused on humans; published in English language; conducted among pregnant women; reported the relevant information including the number or incidence of cases about PTB with GDM women (case group) and normoglycaemic women (control group). The exclusion criteria were: twin or multiple pregnancies; pregnant women with pre-pregnancy diabetes (type 1 or type 2 diabetes); case reports, systematic reviews and meta-analysis articles, studies only included women with GDM; sample size less than or equal to 10 both in case group and control group.
Study selection
Titles and abstracts were screened to identify the potential eligibility for inclusion. Then the selected articles were further analyzed by a full-text review to assess their eligibility for the final inclusion. The reasons for the final inclusion were reviewed by the second author, and disagreements were resolved by further discussion. The articles retrieved from the literature search were screened to eliminate duplicates.
Data extraction and quality assessment
The characteristics of included studies embodied first author’s name, year of publication, country of origin, number of participants, study types, time of the investigation, GDM diagnostic criteria, number of cases with GDM and normal pregnant women. PTB cases in the case group and control group were extracted by two investigators (Wei Li and Jia Zhou). The discrepancies were resolved by discussions with the third author (Mengyao Deng). 9-star Newcastle-Ottawa Quality Assessment Scale was used to evaluate the quality of the included studies, which involved the assessment of three main domains: participant’s selection, comparability, and exposure or outcome [13]. To maximum, a study could be awarded one point for each numbered item within the selection and exposure respectively, and two points for comparability. If the score of the study was up to 6 points or above, the quality of the study would be considered to be good.
Data synthesis and statistical analysis
Heterogeneity between included studies was assessed by the I2 statistics and Q test, and I2>50% or P<0.05 indicated evidence of heterogeneity among studies [14,15]. When substantial heterogeneity was detected, the random-effects model (the DerSimonian-Laird method) was presented for the meta-analysis [16]. Otherwise, the fixed-effects model (the Mantel-Haenszel method) was used.
Sensitivity analyses were performed to see the stability of the results, namely, whether any exclusion of the studies could affect the original results. Subgroup analyses were performed to access the impact of geographical areas and GDM diagnostic criteria on pooled results. Begg’s funnel plot and Egger’s linear regression test were used to assessing possible publication bias. The former was a simple scatter plot of the intervention effect estimates from individual studies against some measure of each study’s size or precision. Formal statistical assessment of funnel plot asymmetry was also incorporated with Egge’s regression asymmetry test and Begg’s adjusted rank correlation test. Statistical tests were conducted by Review Manager Version 5.0 (Windows, Cochrane Collaboration, Oxford, United Kingdom, 2010) and Stata 12.0 (Stata Corporation, College Station, TX, USA). The P value less than 0.05 was considered as statistically significant.
Literature search
Figure 1 performed the flow of study identification and inclusion process. Electronic literature searching yielded 2673 results. Among them, 321 articles were excluded for animal experiments, and 325 were not published in English. There were 2027 articles identified by reading titles and abstracts. Of which, 33 and 120 articles were excluded for case reports, meta-analysis or systematic review respectively, and 1794 articles were excluded on the bias of clear irrelevant. The remaining 80 full-text articles were selected and scrutinized. We excluded 62 articles, which provided no data or did not detect the association between GDM and PTB. Meanwhile, 2 papers were excluded because of duplicate, and 6 articles did not meet the inclusion criteria of this meta-analysis. Finally, there was a total of 10 eligible studies [10,11,17- 24] included in our analysis. In addition, two thresholds were used in Sacks DA’ study [17] to distinguish participants groups, so we regarded the two thresholds as two articles with different GDM diagnostic criteria (IADPSG1, IADPSG2). Hence, there were 11 papers included.
Study characteristics and quality assessment
The main characteristics of the included studies were presented in table 1. In total, the 11 eligible papers which were published from 2001 to 2017 included 2618929 participants (99493 GDM participants and 2531522 No-GDM controls). 7 studies were drawn from North American, 2 studies from Asian and 2 studies from Europe respectively. The quality score of 8 studies met 9 scores, and 2 studies met 7 and 8 scores respectively according to the 9-star Newcastle-Ottawa Scale.
First author’s name | Year of publication |
Number of participants |
Study types | Country of origin | Survey time | GDM group Preterm Total | NO-GDM group Preterm Total | GDM diagnostic criteria |
Quality assessment (points) | ||
Lai FY, et al. [11]. | 2016 | 327198 | Case control | Canada (North America) | 2005-2011 | 1435 | 18137 | 16917 | 306576 | IADPSG 2 | 9 |
Sacks DA, et al. (1) [17]. | 2015 | 9835 | cohort | Southern California (North America) |
2005.10.30- 2010.12.30 |
53 | 771 | 491 | 7943 | IADPSG 1 | 9 |
Sacks DA, et al. (2) [17]. | 2015 | 9835 | cohort |
Southern California (North America) |
2005.10.30- 2010.12.30 |
121 | 1121 | 491 | 7943 | IADPSG 2 | 9 |
Hirst JE, et al. [18]. | 2010 | 2702 | cohort | Vietnam (Asian) |
2010.12.01- 2011.03.31 |
60 | 550 | 141 | 2152 | IADPSG | 9 |
Fadl HE, et al. [19]. | 2010 | 1260297 | cohort | Sweden (Europe) | 1991-2003 | 905 | 10525 | 62489 | 1249772 | DPSG | 9 |
Billionnet C, et al. [20]. | 2017 | 796346 | cohort | France (Europe) | 2012 | 4591 | 57383 | 44475 | 729105 | IADPSG | 9 |
Xiong X, et al. [21]. | 2001 | 111419 | cohort | Canada (North America) | 1991.07.01- 1997.12.31 |
287 | 2755 | 8150 | 108664 | Approximate NDDG | 9 |
Feng H, et al. [22]. | 2017 | 14741 | cohort | China (Asian) |
2013.06.20- 2013.11.30 |
184 | 2927 | 588 | 11814 | IADPSG | 9 |
Boghossian NS, et al. [23]. | 2014 | 62013 | cohort | Utah (North America) | 2002-2010 | 156 | 2275 | 3005 | 58478 | Unclear | 8 |
Hedderson MM, et al. [24]. | 2003 | 46230 | cohort | Northern California (North America) |
1996.01.01- 1998.07.31 |
102 | 1523 | 1541 | 38515 | NDDG | 9 |
Yogev Y, et al. [10]. | 2007 | 12086 | cohort | San Antonio, Texas (North America) |
1995-1999 | 163 | 1526 | 1193 | 10560 | the Carpenter and Coustan Criteria | 7 |
Table 1: Characteristics of the studies that met the inclusion criteria for the meta-analysis.
Synthesis of results
The homogeneity hypothesis was rejected by Q test (χ2=95.01, P<0.001), and the result showed that there was large heterogeneity among studies (I2=89.0%). Therefore, the random-effect model was applied to calculate the pooled OR and the results showed that GDM during pregnancy was associated with a higher risk of PTB (OR=1.43, 95% CI 1.30-1.58) (Figure 2).
Figure 2: Comparison of PTB cases in pregnant women with GDM and in normal pregnant women.
Begg’s funnel plot and Egger’s linear regression test were performed to assess the publication bias of the literature. The shape of the funnel plot did not reveal any sign of significant asymmetry, and the result of Egger’s test also indicated that no evidence of publication bias existed among the studies (P> 0.05). To conduct a sensitivity analysis, we recalculated the combined results by excluding one study per iteration. The results performed that the effect of almost every study included in the pooled estimate was similar in the current meta-analysis.
Subgroup analysis
When stratifying by territory, the included studies were classified as Asian, North American, and European groups. The results indicated that the Asian group (OR=1.45, 95% CI 1.08-1.96), North American group (OR=1.39, 95% CI 1.21-1.59) and European group (OR=1.54, 95% CI 1.16-2.05) all showed an increased risk of PTB with GDM (Figure 3). When stratifying by diagnostic criteria of GDM, these included studies were classified as the NDDG (the National Diabetes Data Group) (fasting, 5.8mmol/l; 1h, 10.6mmol/l; 2h, 9.2mmol/l; 3h, 8.1mmol/l) [25] group, IADPSG1 (fasting, 5.1mmol/l; 1h, 10.0mmol/l; 2h, 8.5mmol/l) [26] group, IADPSG2 (fasting, 5.3mmol/l; 1h, 10.6mmol/l; 2h, 8.9mmol/l) [27] group, and other group (DPSG [28], the Carpenter and Constant Criteria [29]. Similarly, the results indicated that GDM was significantly associated with PTB in the NDDG (OR=1.54, 95% CI 1.29-1.84), IADPSG1 (OR=1.33, 95% CI 1.21-1.46), IADPSG2 (OR=1.60, 95% CI 1.30-1.98) groups (Figure 4).
Figure 3: Forest plot of subgroup analyses of relationships between GDM and PTB in different regions. Events, PTB cases; CI, confidence interval; M-H, Mantel-Haenszel.
Figure 4: Forest plot of subgroup analyses of relationships between GDM and PTB using different diagnostic criteria. Events, PTB cases; CI, confidence interval; M-H, Mantel-Haenszel.
According to the results of our meta-analysis, GDM pregnant women were with 1.43 times higher risk of PTB than women without GDM. Even considering the potential influence of geographical regions and diagnostic criteria, GDM was still a risk factor of PTB. This meant that GDM controlling might reduce the risk of PTB. Popularizing pregnant women to avoid actively the risk factors that trigger GDM during pregnancy might have a significant contribution to the reduction of neonatal PTB rates.
Different regions had different genetic backgrounds and risk models, so we calculated the subgroup analysis by geographical regions. In addition, the GDM diagnostic criteria might also have an effect on the relationship between GDM and PTB. In Sacks DA’s study [17], when GDM was diagnosed by IADPSG2 criteria, there was a significant difference in the incidence of PTB between GDM patients and nonpatients, but this relationship was not found in IADPSG1 group. The IADPSG1 criteria are closer to the normal plasma glucose level than IADPSG2 criteria. So the effect of high blood glucose levels on PTB might be affected by specific concentrations. Perhaps, the higher the concentration was, the stronger the association between GDM and PTB was. The dose-response effect between blood glucose levels and PTB risk require further study.
It should be noted that our meta-analysis results indicated a high heterogeneity both in overall impact and subgroup analyses. From the I2 index formula, we could easily find that to some extent, heterogeneity was affected by the degree of freedom. Nevertheless, the Degree of Freedom (DF) was closely related to the size of the sample. The larger the sample size was, the higher heterogeneity was. In these included studies, Lai FY, et al. [11] and Fadl HE, et al. [19] et al. studies were longterm population-based surveys and these studies sample sizes were more than ten thousand. In addition, although the subgroup analyses were conducted, only the diagnostic criteria of GDM and geographical areas were calculated because of the information limitation. The heterogeneity was also affected by many other confounders such as the age of pregnant women [30], raised body-mass index [31], the history of GDM in a previous pregnancy [23], type of PTB, GDM management and so on. However, because of the differences or deficiencies in the research design of each included article, we could not measure all confounders’ impact on the relationship between GDM and PTB. Different studies had different PTB definitions and classified methods, which might also have an impact on the results of these analyses. Some of our included studies defined PTB as <37 gestational weeks. Some studies defined it as delivery at gestational age <37 weeks and ≥ 28 weeks. On the other hand, some studies divided PTB into spontaneous, indicated, and elective preterm [32,33]. But it was regretted in certain papers. In addition, GDM management protocols might also affect our research results. Good GDM management would help control the glucose content of plasma; even keep blood glucose at normal levels for a long time, so it would have some influence on the pregnancy out come. For example, the results of Bar-Have I’s study [34] showed that blood glucose control in GDM pregnant women would reduce the possibility of PTB.
Although with large sample size and the subgroup analyses by diagnostic criteria for GDM and geographical regions, our results need to be interpreted with caution due to several limitations. Firstly, as mentioned above, we did not identify all sources of heterogeneity and confounders. Secondly, we only considered the impact of GDM, while PTB can be the result of multiple factors, such as maternal hypertension, birth defects and so on. These factors also had an important impact on the overall study outcomes.
In conclusion, the results of our meta-analysis showed that GDM was a risk factor of PTB. On account of the harm of PTB, glycemic control during pregnancy is necessary. However, potential confounders cannot be ruled out completely. Further and in-depth studies are needed to confirm these results.
Acknowledgements
We are very grateful to all the researchers who have provided relevant information for this study.
Funding
This work was supported by Emergency Research Fund for Novel Corona virus Pneumonia Prevention and Control of Wuhan Municipal Health Commission (grant number: EG20A04), Scientific Research Project of Hubei Provincial Health and Family Planning Commission (grant number: WJ2019F011), and Scientific Research Program of Hubei Education Department (grant number: B2020006).
Availability of data and materials
All data pertaining to this study are included in this published article.
Authors’ contributions
W Li: Project development and manuscript reviewing, literature quality assessment, manuscript writing/editing; J Zhou: Literature searching and screening, literature quality assessment, data collection, data analysis, manuscript writing/editing; M Yang: Manuscript reviewing and modification; MY Deng: Literature searching and screening, literature quality assessment, data collection, data analysis, Z Tang: Manuscript reviewing and modification; YGuo: Project development, Idea providing and manuscript reviewing;. All authors read and approved the final manuscript.
Ethics approval and consent to participate
Not applicable.
Consent for publication
Not applicable.
Competing interest
The authors declare that they have no competing interests.
- Metzger BE, Coustan DR (1998) Summary and recommendations of the Fourth International Workshop-Conference on Gestational Diabetes Mellitus. The Organizing Committee. Diabetes Care 21: 161-167. [Ref.]
- Buckley BS, Harreiter J, Damm P, Corcoy R, Chico A, et al. (2012) Gestational diabetes mellitus in Europe: prevalence, current screening practice and barriers to screening. A review. Diabet Med 29: 844-854. [Ref.]
- DeSisto CL, Kim SY, Sharma AJ (2014) Prevalence estimates of gestational diabetes mellitus in the United States, Pregnancy Risk Assessment Monitoring System (PRAMS), 2007-2010. Prev Chronic Dis 11: E104. [Ref.]
- Zhu WW, Yang HX, Wei YM, Yan J, Wang ZL, et al. (2013) Evaluation of the value of fasting plasma glucose in the first prenatal visit to diagnose gestational diabetes mellitus in china. Diabetes Care 36: 586-590. [Ref.]
- Davey RX (2005) Gestational diabetes mellitus: a review from 2005. Curr Diabetes Rev 1: 203-213. [Ref.]
- Goldenberg RL, Culhane JF, Iams JD, Romero R (2008) Epidemiology and causes of preterm birth. Lancet 371: 75-84. [Ref.]
- Berkowitz GS, Papiernik E (1993) Epidemiology of preterm birth. Epidemiol Rev 15: 414-443. [Ref.]
- Liu L, Johnson HL, Cousens S, Perin J, Scott S, et al. (2012) Global, regional, and national causes of child mortality: an updated systematic analysis for 2010 with time trends since 2000. Lancet 379: 2151-2161. [Ref.]
- Mwaniki MK, Atieno M, Lawn JE, Newton CR (2012) Long-term neurodevelopmental outcomes after intrauterine and neonatal insults: a systematic review. Lancet 379: 445-452. [Ref.]
- Yogev Y, Langer O (2007) Spontaneous preterm delivery and gestational diabetes: the impact of glycemic control. Arch Gynecol Obstet 276: 361-365. [Ref.]
- Lai FY, Johnson JA, Dover D, Kaul P (2016) Outcomes of singleton and twin pregnancies complicated by pre-existing diabetes and gestational diabetes: A population-based study in Alberta, Canada, 2005-11. J Diabetes 8: 45-55. [Ref.]
- Moher D, Liberati A, Tetzlaff J, Altman DG, PRISMA Group (2010) Preferred reporting items for systematic reviews and meta-analyses: the PRISMA statement. Int J Surg 8: 336-341. [Ref.]
- Wells GA, Shea B, Connell DO, Peterson J, Welch V, et al. The Newcastle-Ottawa Scale (NOS) for assessing the quality of nonrandomised studies in meta-analyses. [Ref.]
- Higgins JPT, Green S, Cochrane Collaboration (2008) Cochrane handbook for systematic reviews of interventions. Wiley, England: 649. [Ref.]
- Higgins JP, Thompson SG (2002) Quantifying heterogeneity in a meta-analysis. Stat Med 21: 1539-1558. [Ref.]
- DerSimonian R, Laird N (2015) Meta-analysis in clinical trials revisited. Contemp Clin Trials 45: 139-145. [Ref.]
- Sacks DA, Black MH, Li X, Montoro MN, Lawrence JM (2015) Adverse Pregnancy Outcomes Using The International Association of the Diabetes and Pregnancy Study Groups Criteria: Glycemic Thresholds and Associated Risks. Obstet Gynecol 126: 67-73. [Ref.]
- Hirst JE, Tran TS, Do MA, Morris JM, Jeffery HE (2012) Consequences of gestational diabetes in an urban hospital in Viet Nam: a prospective cohort study. PLoS Med 9: e1001272. [Ref.]
- Fadl HE, Ostlund IK, Magnuson AF, Hanson US (2010) Maternal and neonatal outcomes and time trends of gestational diabetes mellitus in Sweden from 1991 to 2003. Diabet Med 27: 436-441. [Ref.]
- Billionnet C, Mitanchez D, Weill A, Nizard J, Alla F, et al. (2017) Gestational diabetes and adverse perinatal outcomes from 716,152 births in France in 2012. Diabetologia 60: 636-644. [Ref.]
- Xiong X, Saunders LD, Wang FL, Demianczuk NN (2001) Gestational diabetes mellitus: prevalence, risk factors, maternal and infant outcomes. Int J Gynaecol Obstet 75: 221-228. [Ref.]
- Feng H, Zhu WW, Yang HX, Wei YM, Wang C, et al. (2017) Relationship between Oral Glucose Tolerance Test Characteristics and Adverse Pregnancy Outcomes among Women with Gestational Diabetes Mellitus. Chin Med J (Engl) 130: 1012-1018. [Ref.]
- Boghossian NS, Yeung E, Albert PS, Mendola P, Laughon, et al. (2014) Changes in diabetes status between pregnancies and impact on subsequent newborn outcomes. Am J Obstet Gynecol 210: 431.e1- e14. [Ref.]
- Hedderson MM, Ferrara A, Sacks DA (2003) Gestational diabetes mellitus and lesser degrees of pregnancy hyperglycemia: association with increased risk of spontaneous preterm birth. Obstet Gynecol 102: 850-856. [Ref.]
- (1979) Classification and diagnosis of diabetes mellitus and other categories of glucose intolerance. National Diabetes Data Group. Diabetes 28: 1039-1057. [Ref.]
- International Association of Diabetes and Pregnancy Study Groups Consensus Panel, Metzger BE, Gabbe SG, Persson B, Catalano PA, et al. (2010) International association of diabetes and pregnancy study groups recommendations on the diagnosis and classification of hyperglycemia in pregnancy. Diabetes Care 33: 676-682. [Ref.]
- Canadian Diabetes Association Clinical Practice Guidelines Expert Committee, Thompson D, Berger H, Feig D, Gagnon R, et al. (2013) Diabetes and pregnancy. Can J Diabetes 37: 168-183. [Ref.]
- Anderberg E, Källén K, Berntorp K, Frid A, Aberg A (2007) A simplified oral glucose tolerance test in pregnancy: compliance and results. Acta Obstet Gynecol Scand 86: 1432-1436. [Ref.]
- Carpenter MW, Coustan DR (1982) Criteria for screening tests for gestational diabetes. Am J Obstet Gynecol 144: 768-773. [Ref.]
- Lamminpää R, Vehviläinen-Julkunen K, Gissler M, Selander T, Heinonen S (2014) Pregnancy outcomes in women aged 35 years or older with gestational diabetes - a registry-based study in Finland. J Matern Fetal Neonatal Med 29: 55-59. [Ref.]
- Martino J, Sebert S, Segura MT, García-Valdés L, Florido J, et al. (2016) Maternal Body Weight and Gestational Diabetes Differentially Influence Placental and Pregnancy Outcomes. J Clin Endocrinol Metab 101: 59-68. [Ref.]
- Laughon SK, Reddy UM, Sun L, Zhang J (2010) Precursors for late preterm birth in singleton gestations. Obstet Gynecol 116: 1047- 1055. [Ref.]
- Laughon SK, Albert PS, Leishear K, Mendola P (2014) The NICHD Consecutive Pregnancies Study: recurrent preterm delivery by subtype. Am J Obstet Gynecol 210: 131. [Ref.]
- Bar-Hava I, Barnhard Y, Scarpelli SA, Orvieto R, Ben-Rafael, et al. (1997) Gestational diabetes and preterm labour: is glycaemic control a contributing factor? Eur J Obstet Gynecol Reprod Biol 73: 111-114. [Ref.]
Download Provisional PDF Here
Article Type: REVIEW ARTICLE
Citation: Li W, Zhou J, Yang M, Deng M, Tang Z, et al. (2021) Relationship between Gestational Diabetes Mellitus and Preterm Birth: a Meta-analysis. Int J Endocrinol Metab Disord 7(1): dx.doi.org/10.16966/2380-548X.176
Copyright: © 2021 Li W, et al. This is an open-access article distributed under the terms of the Creative Commons Attribution License, which permits unrestricted use, distribution, and reproduction in any medium, provided the original author and source are credited.
Publication history:
SCI FORSCHEN JOURNALS
All Sci Forschen Journals are Open Access